Machine Learning
Machine learning potentials
We combine machine learning (ML) with quantum mechanics and molecular dynamics to efficiently study conformational flexibility and solvent effects in organic and organometallic reactions.
Machine learning for molecular design
We apply a range of machine learning models to predict chemical properties and guide the design of new synthetic pathways. We are particularly interested in interpreting our models’ predictions.
Relevant Publications
S. Zavitsanou, Z. Bo, E. Casali, M. Langton, and F. Duarte. PythiaCHEM : a user-friendly machine learning toolkit for chemistry, ChemRxiv 2024 Preprint
T. A. Young, T. Johnston-Wood, H. Zhang, F. Duarte. Reaction dynamics of Diels–Alder reactions from machine learned potentials. Phys. Chem. Chem. Phys., 2022, 24, 20820–20827.
T. A. Young, T. Johnston-Wood, V. Deringer, F. Duarte. A Transferable Active-Learning Strategy for Reactive Molecular Force Fields. Chem. Sci., 2021, 12, 10944.
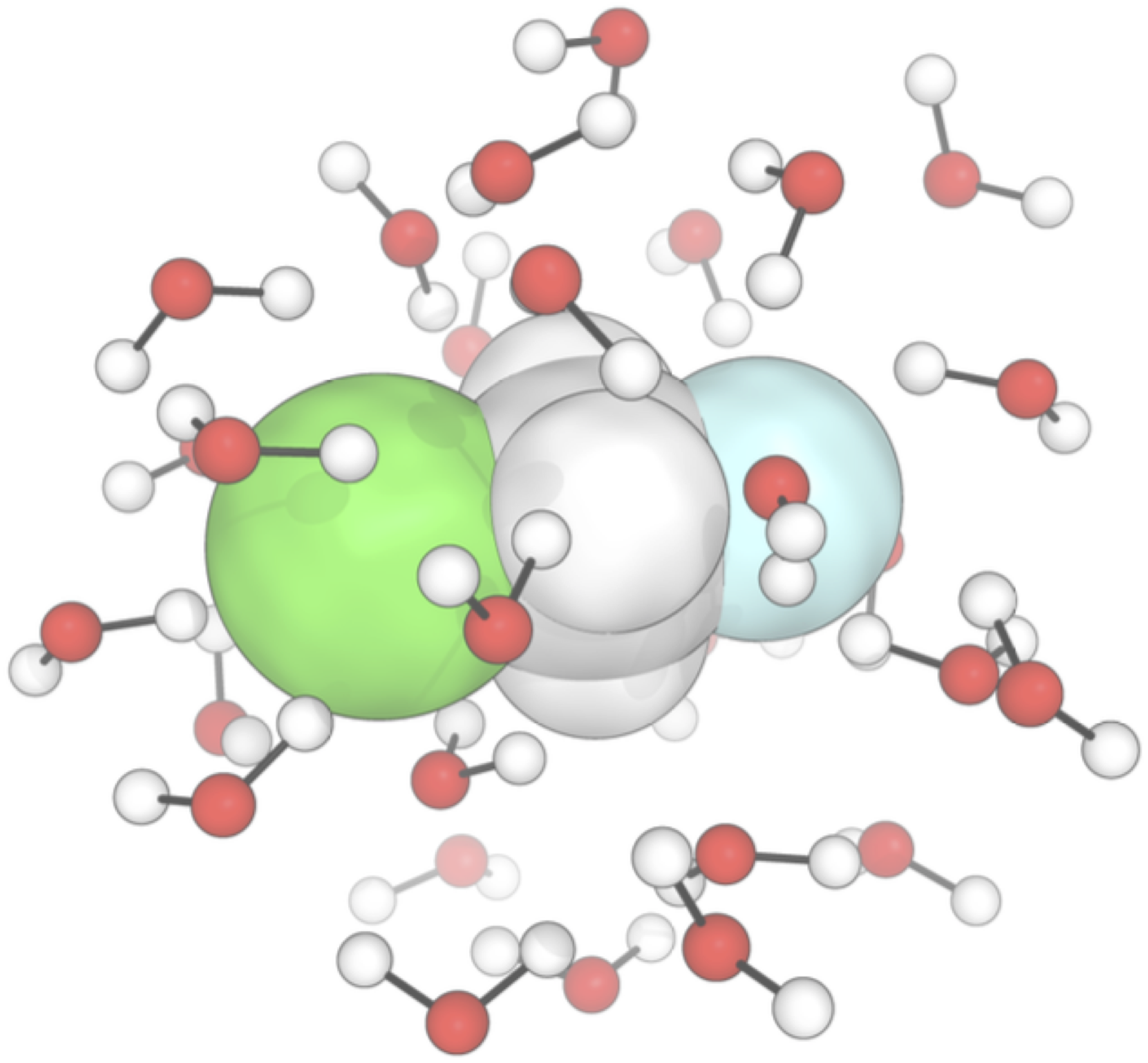